There are two main methods when it comes to implementing a health score for your SaaS software. While the business-rules-based historical approach has several limitations, the newer AI-based models are more reliable and significantly faster to deploy. Explanations.
Challenges with the implementation of a "classic" health score
First generation health scores work with business rules, meaning that it is up to the user to define the conditions that make a customer "healthy" or "unhealthy". These are rules like "if I have at least N users who use feature X in the Y days following [step A] AND [new condition], THEN ...".
This may eventually work for some companies that are very mature about measuring the health of their accounts, but for most SaaS vendors, it poses at least 3 problems:
- First, you are asked to define the rules for predicting churn. Except that, most of the time, you don't know! Simply because you don't have an army of Data-Scientists who have analyzed for you the customer data that preceded your churns. In other words, you probably have your business preconceptions, but no reliable data to confirm your assumptions. But, as W. Edwards Deming, "without data, you are just another person with an opinion".
- Each business rule uses a small set of data, since by definition you define - and limit - the criteria to be considered. So traditional health scores are usually based on a few metrics that you think are important... and leave the other criteria out. You can miss out on essential criteria for many months. Until the day a customer who was supposed to be healthy sends you a termination letter. Then, you will analyze his behavior in more detail and will indeed find abnormal data... but that was not considered in your health score. Most companies who have implemented health score like this tell that it takes several iterations before you have a fairly reliable score. The question is: how much churn will you have in the meantime?
- Finally, these scores are based on fixed criteria. However, your product is getting richer, perhaps your customer typology and your commercial offer are evolving. In short, each change must be manually reflected in your score... otherwise you risk having a rapidly obsolete model. You must therefore plan resources to maintain and develop this type of health score. Profiles with a technical background may be necessary, as the scores are sometimes similar to real SQL queries!
The answers provided by Artificial Intelligence
An AI like Skalin's allows to answer efficiently to all these challenges by relying on 3 fundamental principles:
#1. Comparative analysis of your clients, without preconceived ideas
Our AI continuously analyzes more than 70 KPIs across your entire portfolio to identify customers at risk and detect those with potential.
These indicators are divided into 4 main categories:
- Customer conversations: do you regularly exchange with your customer? what is your "footprint" on the account (are you rather dependent on one person or do you have several entry points, are you in control of the decision-maker...)? what is the level of engagement of your contacts (emails response rate, decision-maker attending QBRs...). In addition, sentiment analysis allows you to extract the general state of mind of your contacts thanks to the study of the emails/tickets exchanged, and this in all main business languages. Thus, a contact that is not going well will be automatically detected. And if this happens more often to this customer than to others, his health score will be impacted.
- Product usage: how often do your customers log in? what is the ration of active users? how does their connection rate evolve? how much time do they spend on your platform? do they use the key features of your tools or are they just using them superficially?
- Revenue data: has your customer been loyal to you for a long time? is the value of their contract increasing or descreasing? are they consuming additional services? is the contract due to expire soon or in the distant future? what is their financial health level?
- CSM Pulse, i.e. the opinion of the CSM itself on its accounts.
These metrics are calculated for each customer segment, which automatically identifies accounts that are weak on a metric, compared to their peer group. A customer who is weak on too many KPIs will automatically see their health score drop. So you don't have to worry about defining the criteria to analyze, the AI does it for you! And it does it fast.
#2. Prediction of future behavior
Taking into account the evolution of each KPIs makes it possible to nuance the previous analysis. For example, a customer may have a low connection frequency compared to his segment, but if he progresses compared to his own history, the AI will take this into account and consider that the customer is in a positive dynamic. The analysis of the history allows to refine the calculation of the health score and to predict the future behavior of a customer.
#3. Self-learning model
While the first point allows AI to be effective very quickly, the integration of Machine Learning models optimizes the score over time. When a customer churns or becomes inactive for a long time, the AI will integrate previous behaviors in its model to progressively give more weight to the most discriminating variables. The more data history the AI has, the more relevant it will be.
What place for personalization?
AI can also be used to customize a health score by weighting important criteria for each customer segment, or by integrating custom metrics.
Weighted criteria specifically for each customer segment
You can define the weight you want to give to each category of criteria and even have specific weightings for each customer typology. For example, your "High-Touch" customers will have a health score that is more influenced by interactions and CSM Pulse, while your "Low-Touch" customers will be scored mainly on their product usage.
Taking Custom Metrics into account
Not all relevant metrics can be tracked directly on your application. This is where "Custom Metrics" come in. They allow you to send additional information to the algorithm in order to adjust the score up or down. Let's say you sell a marketing activation solution: it's certainly important to know if your users are logging in regularly and using your key features. But if they don't get any ROI from it, then the relationship isn't going to last. It is therefore possible to inject business criteria (ROI, leads generated...) to enrich your health score.
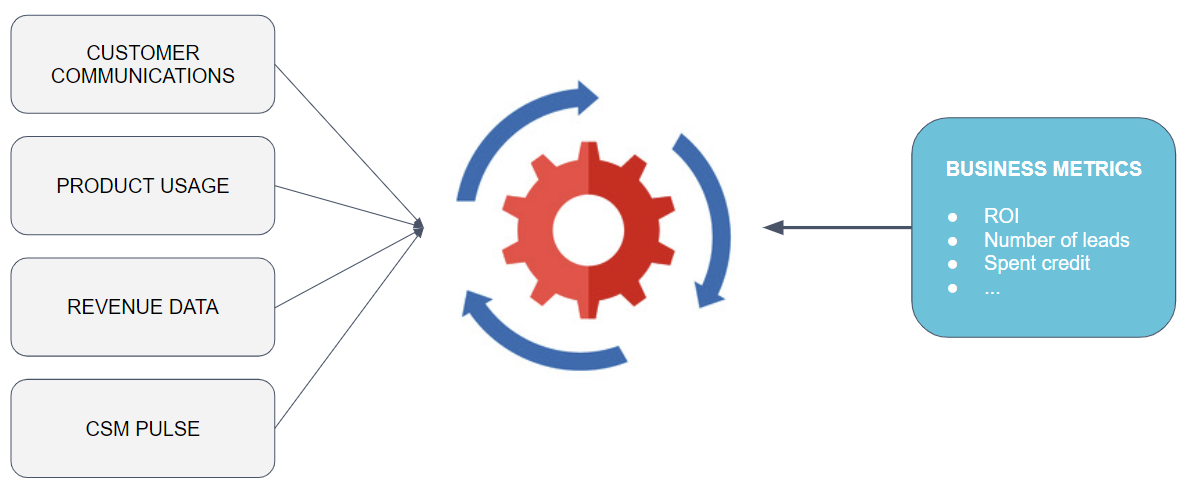
All this in a UI 100% manageable by the business of course!
AI helps deploy a Health Score faster
AI can analyze an impressive variety of factors: more than 70 criteria analyzed from D-1, globally and by customer segment, continuously historicized, predicting future behaviors... it is way more powerful than any manually managed score. And yet it is so easy to implement!
Because beyond pure performance, setting up an AI-based health score is also much faster than setting up a score based on business rules. And for good reason! Because it is the AI that natively interprets the vital metrics of your customers. In terms of configuration, setting up a health score is Plug&Play, so to speak. However, it is estimated that 2 to 3 weeks of usage data are needed before the score is really relevant. In short, in less than a month you have an operational and reliable health score.
Would you like to know more? Do not hesitate to make an appointment with our experts